Target and Organs-at-Risk Segmentation
Accurate and timely target and organs-at-risk (OAR) segmentation is key to efficient and high-quality radiation therapy planning. Manual segmentation is time-consuming and subject to inter- and intra-observer variation. Deep learning-based methods have been applied into medical image segmentation tasks, especially tumor and multi-OAR segmentation.
Related publication(s):
Fu Y, Lei Y, Wang T, Curran W, Liu T and Yang X*. ‘A Review of Deep Learning based Methods for Medical Image Multi-Organ Segmentation,” European Journal of Medical Physics (Physica Medica), 85, 107-122, 2021.
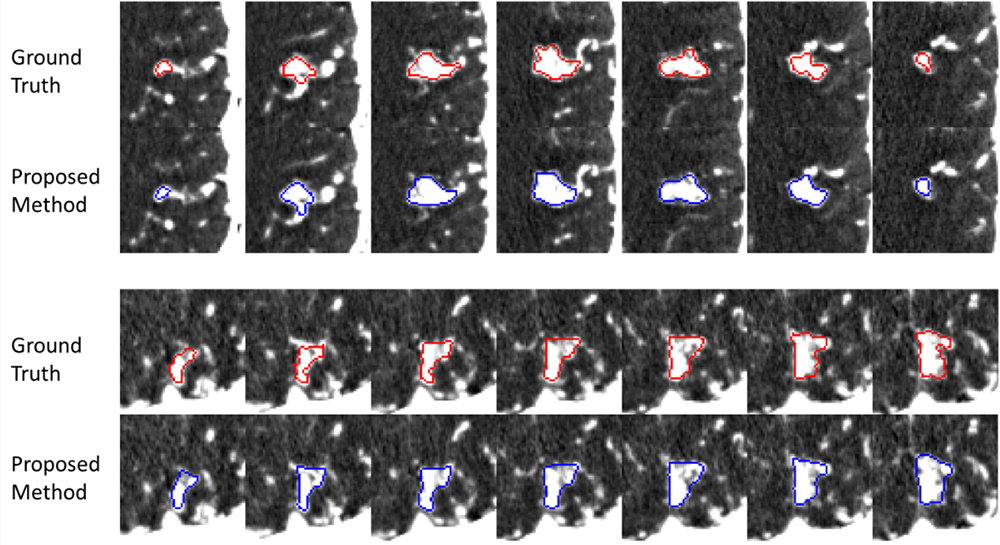
Brain
Related publications:
Wang T, Lei Y, Tian S, Jiang X, Zhou J, Dresser S, Walter C, Shu K, Yang X*. “Learning-based Automatic Segmentation of Arteriovenous Malformations on Contrast CT Images in Brain Stereotactic Radiosurgery,” Medical Physics, 46(5):3133-3141, 2019.
Lei Y, Tian Z, Kahn S, Curran W, Liu T and Yang X*. "Automatic Detection of Brain Metastases using 3D Mask R-CNN for Stereotactic Radiosurgery," Proc. of SPIE, 11314, 113142X-9X, 2020.
Jeong J, Lei Y, Kahn S, Liu T, Curran W, Shu H, Mao H and Yang X*. “Brain Tumor Segmentation Using 3D Mask R-CNN for Dynamic Susceptibility Contrast Enhanced Perfusion Imaging,” Physics in Medicine and Biology, 65(18):185009, 2020.
Lin M, Momin S, Lei Y, Wang H, Curran WJ, Liu T, Yang X*. “Fully Automated Segmentation of Brain Tumor from Multiparametric MRI Using 3D Context Deep Supervised U-Net,” Medical Physics, 48(8):4365-4374, 2021.
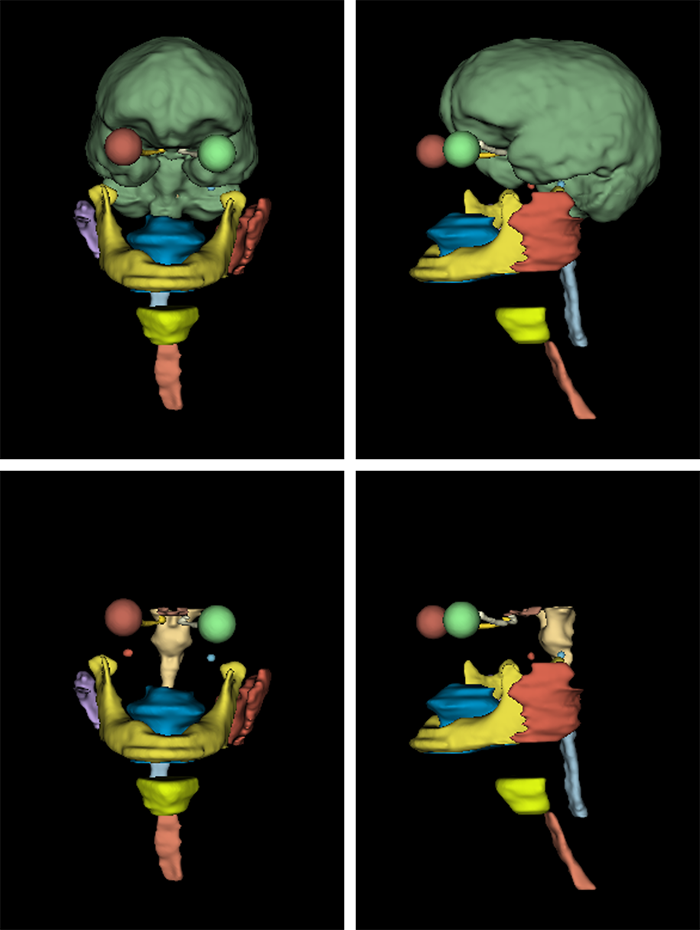
Head and Neck
Related publications:
Lei Y, Zhou J, Dong X, Wang T, Mao H, McDonald M, Curran W, Liu T and Yang X*. "Multi-Organ Segmentation in Head and Neck MRI Using U-Faster-RCNN," Proc. of SPIE, 11313, 113133A-9A, 2020.
Lei Y, Harms J, Dong X, Wang T, Tang X, Yu D, Beitler J, Curran W, Liu T and Yang X*. "Organ-at-Risk (OAR) Segmentation in Head and Neck CT Using U-RCNN," Proc. of SPIE, 11314, 1131444-51, 2020.
Liu Y, Lei Y, Fu Y, Wang T, Zhou J, Jiang X, McDonald M, Beitler J, Curran W, Liu T and Yang X*. “Head and Neck Multi-Organ Auto-Segmentation on CT Images Aided by Synthetic MRI," Medical Physics, 47(9):4294-4302, 2020.
Dai X, Lei Y, Wang T, McDonald M, Beilter J, Curran W, Zhou J, Liu T and Yang X*. “Synthetic MRI-aided Head-and-Neck Organs-at-Risk Auto-Delineation for CBCT-guided Adaptive Radiotherapy,” Physics in Medicine and Biology, 66(4):045021, 2021.
Wang T, Lei Y, Roper J, Ghavidel B, Beitler JJ, McDonald M, Curran WJ, Liu T and Yang X*. “Head and neck multi-organ segmentation on dual-energy CT using dual pyramid convolutional neural networks.” Physics in Medicine and Biology, 66 (11), 115008, 2021.
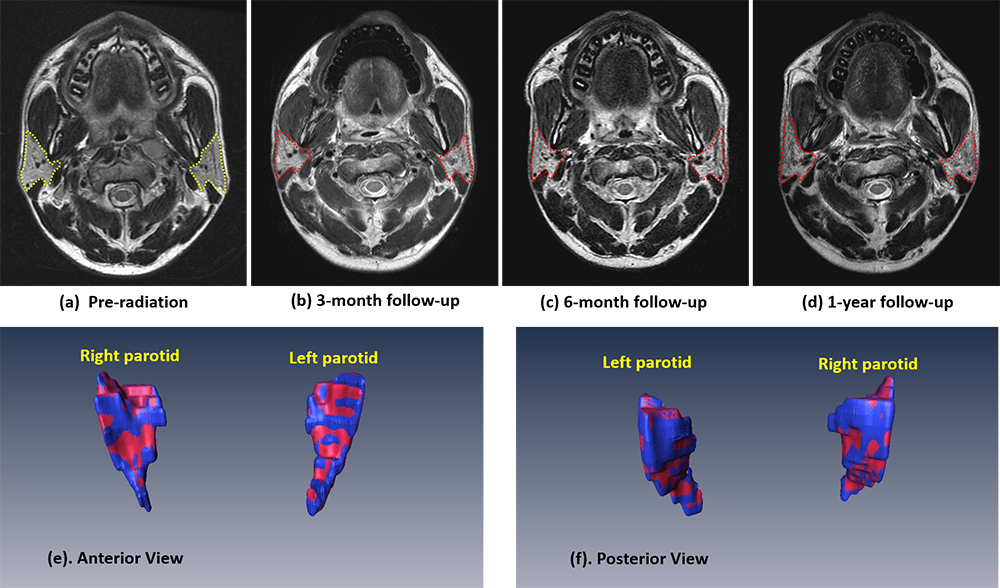
Parotid
Related publications:
Yang X, Wu N, Cheng G, Zhou Z, Yu D, Beitler J, Curran W and Liu T, " Automated Segmentation of the Parotid Gland Based on Atlas Registration and Machine Learning: A Longitudinal MRI Study in Head-And-Neck Radiation Therapy.” International Journal of Radiation Oncology • Biology • Physics (IJROBP), 90(5), 1225-1233, 2014.
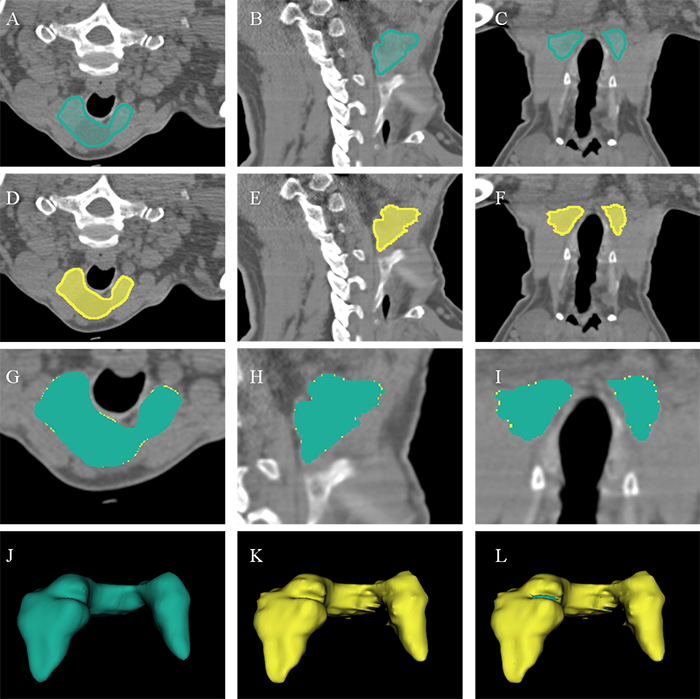
Thyroid
Related publications:
He X, Guo B, Lei Y, Tian S, Wang H, Curran W, Zhang L, Liu T and Yang X*. “Thyroid Gland Delineation in Non-contrast-enhanced CT Using Deep Convolutional Neural Networks: A Cross-sectional Study,” Physics in Medicine and Biology, 66(5), 055007, 2021.
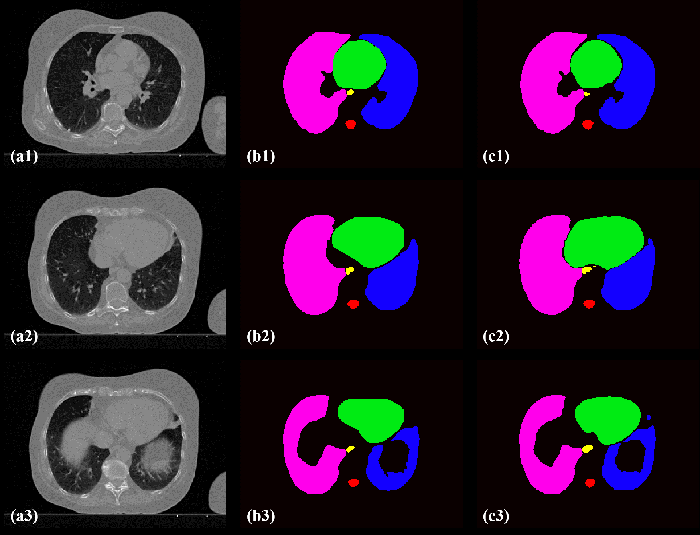
Chest
Related publications:
Dong X, Lei Y, Wang T, Thomas M, Tang L, Curran W, Liu T and Yang X*. “Automatic Multi-Organ Segmentation in Thorax CT Images Using U-Net-GAN," Medical Physics, 46(5):2157-2168, 2019. (Editor’s Choice)
Momin S, Lei Y, Tian Z, Wang T, Roper J, Kesarwala A, Higgins K, Bradley j, Liu T and Yang X*. “Lung Tumor Segmentation in 4D CT Images Using Motion Convolutional Neural Networks,” Medical Physics, 2021. (Accepted)
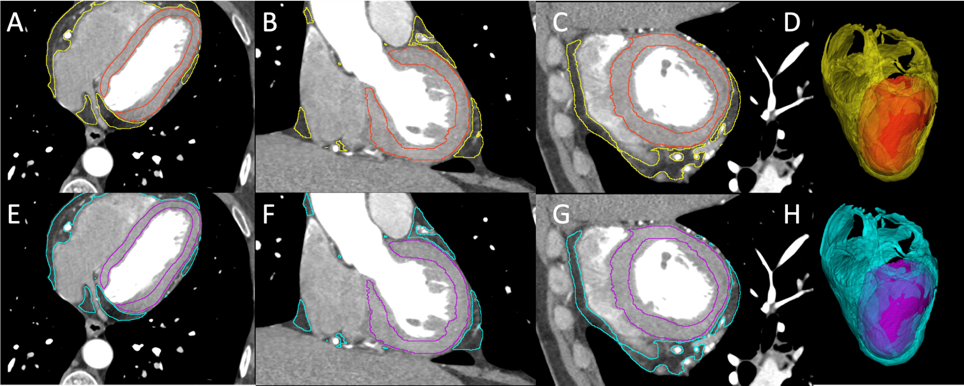
Heart
Related publications:
Wang T, Lei Y, Tang H, He Z, Castillo R, Wang C, Li D, Higgins K, Liu T, Curran W, Zhou W* and Yang X*. “A Learning-based Automatic Segmentation and Quantification Method on Left Ventricle in Gated Myocardial Perfusion SPECT Imaging: A Feasibility Study," Journal Nuclear Cardiology, 1-12, 2019.
Guo B, He X, Lei Y, Harms J, Wang T, Curran W, Liu T, Zhang L and Yang X*. “Automated Left Ventricular Myocardium Segmentation Using 3D Deeply Supervised Attention U-Net for Coronary Computed Tomography Angiography,” Medical Physics, 47(4):1775-1785, 2020.
He X, Guo B, Lei Y, Wang T, Fu Y, Curran W, Zhang L, Liu T* and Yang X*. "Automatic Segmentation and Quantification of Epicardial Adipose Tissue from Coronary Computed Tomography Angiography," Physics in Medicine and Biology, 65(9):095012, 2020.
He X, Guo B, Lei Y, Wang H, Curran W, Zhang L, Liu T and Yang X*. “Automatic Quantification of Left Ventricle Myocardium and Epicardial Adipose Tissue from Coronary Computed Tomography Angiography: A Multicenter Study,” European Radiology, 31 (6), 3826-3836, 2021.
Lei Y, Fu Y, Justin R, Kristin H, Curran W, Liu T and Yang X*. “Echocardiographic Image Multi-Structure Segmentation using Cardiac-SegNet,” Medical Physics, 48(5): 2426-2437 2021.
Harms J, Lei Y, Tian S, McCall N, Higgins K, Bradley J, Curran WJ, Liu T, Yang X*. “Automatic Delineation of Cardiac Substructures using a Region-Based Fully Convolutional Network.” Medical Physics, 48(6):2867-2876, 2021.
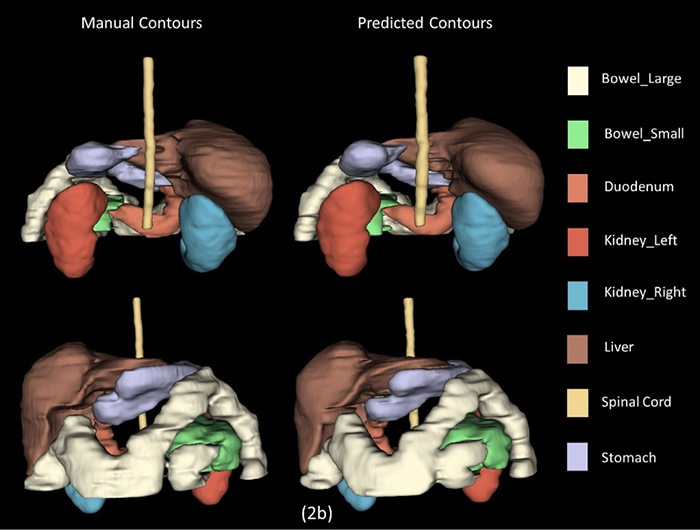
Abdomen
Related publications:
Liu Y, Lei Y, Fu Y, Wang T, Tang X, Jiang X, Curran W, Liu T, Patel P and Yang X*. “CT-based Multi-Organ Segmentation Using a Deep-learning-based Network for Pancreatic Radiotherapy,” Medical Physics, 47(9), 4316-4324, 2020.
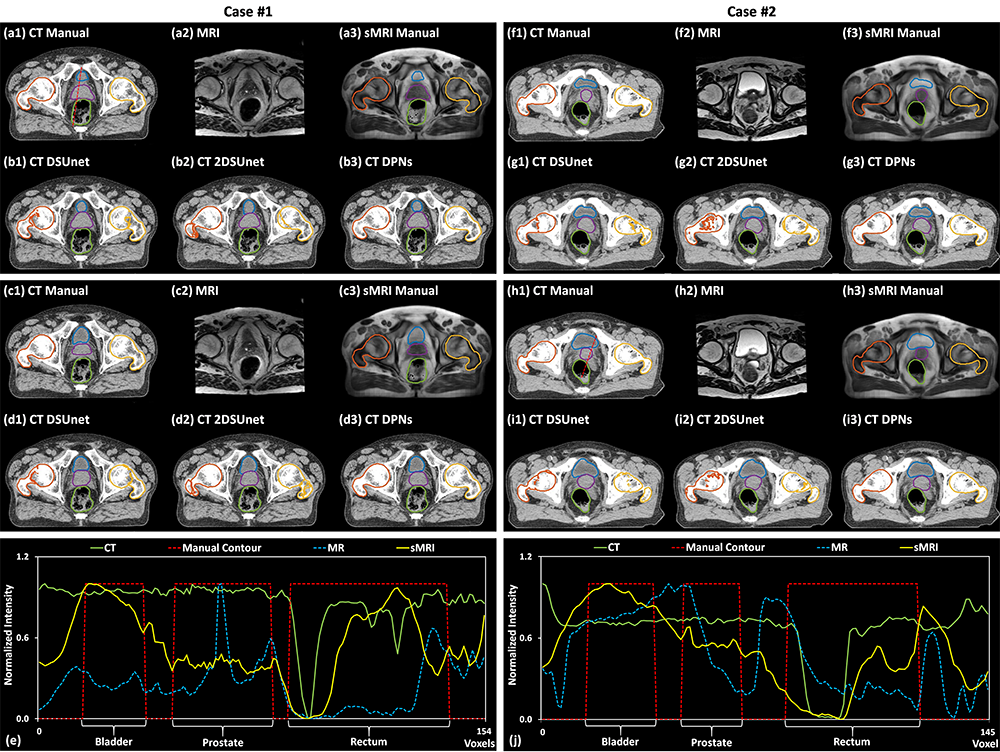
Pelvis
Related publications:
Zhang Y, He X, Lei Y, Wang T, Mao H, Jani A, Patel P, Curran W, Liu T and Yang X*. “Multi-needle Detection in 3D Ultrasound Images Using Unsupervised Order-graph Regularized Sparse Dictionary Learning,” IEEE Transactions on Medical Imaging, 39(7), 2302-2315, 2020.
Dong X, Lei Y, Liu Y, Wang T, Tian S, Jani A, Patel P, Shuster D, Curran W, Liu T and Yang X*. “Synthetic MRI-aided multi-organ segmentation on male pelvic CT using cycle consistent deep attention network,” Radiotherapy and Oncology, 141, 192-199 2019.
Wang B, Lei Y, Tian S, Wang T, Liu Y, Pretesh P, Jani A, Mao H, Curran W, Liu T and Yang X*. “Deeply Supervised 3D FCN with Group Dilated Convolution for Automatic MRI Prostate Segmentation" Medical Physics, 46(4):1707-1718, 2019.
Lei Y, Tian S, He X, Wang T, Wang B, Pretesh P, Jani A, Mao H, Curran W, Liu T and Yang X*. “Ultrasound Prostate Segmentation Based on Multi-Directional Deeply Supervised V-Net," Medical Physics, 46(6):3194-3206, 2019.
Lei Y, Dong X, Tian Z, Liu Y, Wang T, Tian S, Patel P, Jani A, Curran W, Mao H, Liu T and Yang X*. “CT Prostate Segmentation Based on Synthetic MRI-aided Deep Attention Fully Convolution Network,” Medical Physics, 47(1), 530-540, 2020.
Lei Y, Wang T, Tian S, Dong X, Jani A, Schuster D, Curran W, Patel P, Liu T and Yang X*. “Male Pelvic Multi-Organ Segmentation Aided by CBCT-based Synthetic MRI,” Physics in Medicine and Biology, 65(3), 035013, 2020.
Fu Y, Lei Y, Fu Y, Wang T, Tian S, Patel P, Jani A, Curran W, Liu T and Yang X*. “Pelvic Multi-organ Segmentation on CBCT for Prostate Adaptive Radiotherapy," Medical Physics, 47(8):3415-3422, 2020.
Zhang Y, Lei Y, Qiu R, Wang T, Wang H, Jani A, Curran W, Patel P, Liu T and Yang X*. “Multi-needle Localization with Total Variation Regularized Deep Supervised Attention U-Net in Ultrasound-guided HDR Prostate Brachytherapy," Medical Physics, 47(7):2735-2745, 2020.
Lei Y, Wang T, Tian S, Fu Y, Patel P, Jani A, Curran W, Liu T and Yang X*. “Male Pelvic CT Multi-organ Segmentation using Synthetic MRI-aided Dual Pyramid Networks,” Physics in Medicine and Biology, 66(8): 085007 2021.
Yang X, Rossi P, Jani A, Mao H, Ogunleye T, Curran W and Liu T. “3D Transrectal Ultrasound (TRUS) Prostate Segmentation Based on Optimal Feature Learning Framework.” Proc. of SPIE, 97842F-97842F-7, 2016.
Yang X, Jani A, Rossi P, Mao H, Curran W and Liu T. “Patch-Based Label Fusion for Automatic Multi-atlas-based Prostate Segmentation in MR Images.” Proc. of SPIE, 978621-978621-7, 2016.
Yang X, Rossi P, Ogunleye T, Marcus D, Jani A, Mao H, Curran W and Liu T. “Prostate CT Segmentation Method Based on Deformable Registration in Ultrasound-Guided CT-Based HDR Prostate Brachytherapy.” Medical Physics, 41(11), 111915, 2014.
Yang X*, Rossi, P, Jani A, Mao H, Ogunleye T, Curran W and Liu T*. "Improved prostate delineation in prostate HDR brachytherapy with TRUS-CT deformable registration technology: A pilot study with MRI validation.” Journal of Applied Clinical Medical Physics, 45 (2), 440-449, 2017.
Deformable Image Registration
Image registration, also known as image fusion or image matching, is the process of aligning two or more images based on image appearances. Medical image registration seeks to find an optimal spatial transformation that best aligns the underlying anatomical structures. Medical image registration is used in many clinical applications such as image-guided intervention, motion tracking, segmentation, dose accumulation, image reconstruction and so on.
Related publications:
Fu Y, Lei Y, Wang T, Curran W, Liu T and Yang X*. “Deep Learning in Medical Image Registration: A Review,” Physics in Medicine and Biology, 65 (20), 20TR01, 2020.
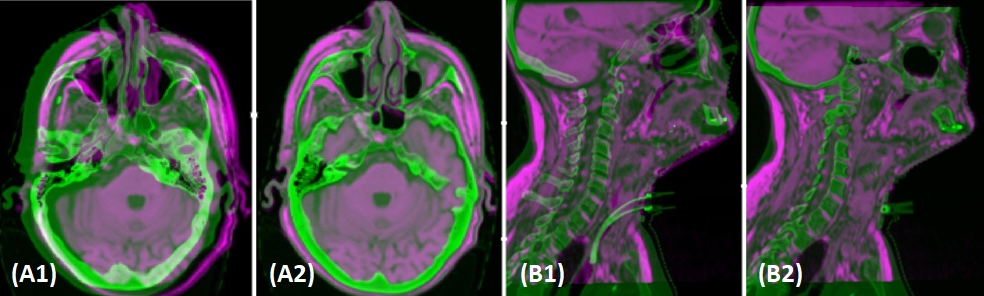
Head and Neck: MRI-CT
Related publications:
Fu Y, Lei Y, Zhou J, Wang T, Yu D, Beitler J, Curran W, Liu T and Yang X*. "Synthetic CT-aided MRI-CT Image Registration for Head and neck Radiotherapy," Proc. of SPIE, 11313, 113132U-9U, 2020.
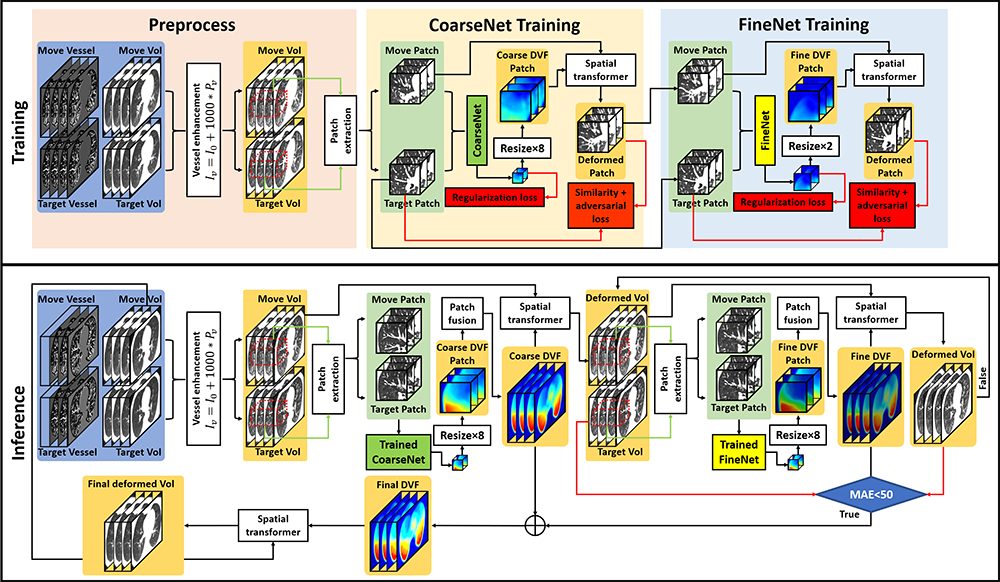
Lung: 4D CT
Deformable image registration of 4D-CT images is important in multiple radiation therapy applications including motion tracking of soft tissue or fiducial markers, target definition, image fusion, dose accumulation and treatment response evaluations.
Related publications:
Fu Y, Lei Y, Wang T, Liu Y, Higgins K, Bradley J, Curran W, Liu T and Yang X*. “LungRegNet: An Unsupervised Deformable Image Registration Method for 4D-CT Lung,” Medical Physics, 2020. 47 (4):1763-1774, 2020. (Editor’s Choice)
Momin S, Lei Y, Tian Z, Wang T, Roper J, Kesarwala A, Higgins K, Bradley j, Liu T and Yang X*. “Lung Tumor Segmentation in 4D CT Images Using Motion Convolutional Neural Networks,” Medical Physics, 2021. (In press)
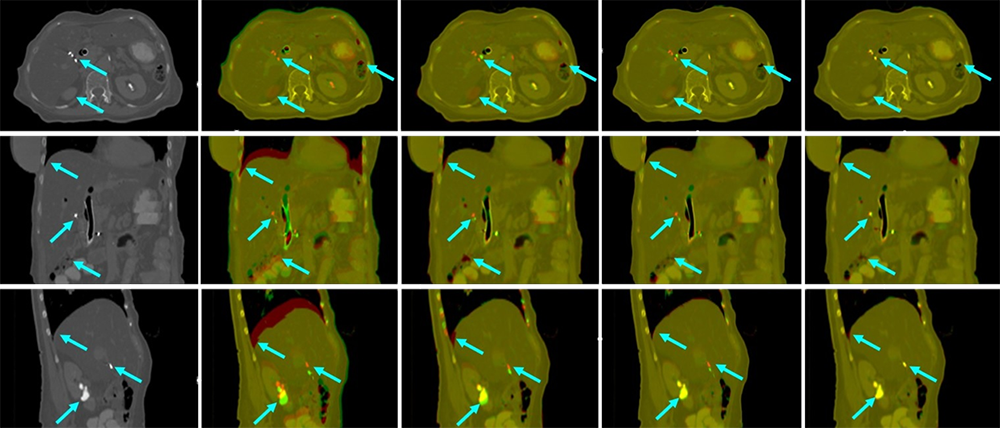
Abdomen: 4D CT
Related publications:
Lei Y, Fu Y, Wang T, Liu Y, Higgins K, Curran W, Liu T and Yang X*. “4D-CT Deformable Image Registration Using Multiscale Unsupervised Deep Learning,” Physics in Medicine and Biology, 65(8), 085003, 2020.
Lei Y, Fu Y, Harms J, Wang T, Curran W, Liu T, Higgins K and Yang X*. 4D-CT Deformable Image Registration Using an Unsupervised Deep Convolutional Neural Network. In: Nguyen D., Xing L., Jiang S. (eds) Artificial Intelligence in Radiation Therapy. AIRT 2019. Lecture Notes in Computer Science, vol 11850. Springer, Cham.
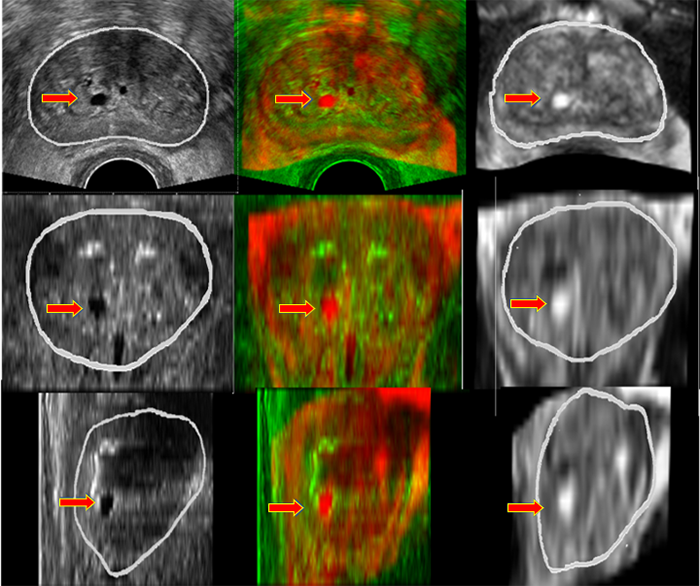
Pelvis: MRI-CT, MRI-US, CT-CBCT and MRI-CBCT
Related publications:
Zeng Q, Fu Y, Tian Z, Lei Y, Zhang Y, Wang T, Wang H, Mao H, Liu T, Curran W, Jani A, Patel P and Yang X*. “Label-Driven MRI-US Registration Using Weakly-Supervised Learning for MRI-guided Prostate Radiotherapy," Physics in Medicine and Biology, 65(13):135002, 2020.
Fu Y, Lei Y, Wang T, Patel P, Jani A, Mao H, Curran W, Liu T and Yang X*. "Biomechanically Constrained Non-rigid MR-TRUS Prostate Registration using Deep Learning based 3D Point Cloud Matching," Medical Image Analysis, 67,101845, 2021.
Fu Y, Wang T, Lei Y, Patel P, Jani A, Curran W, Liu T and Yang X*. “Deformable MR-CBCT Prostate Registration using Biomechanically Constrained Deep Learning Networks,” Medical Physics, 48(1):253-263, 2021.
Lei Y, Wang T, Tian S, Dong X, Jani A, Schuster D, Curran W, Patel P, Liu T and Yang X*. "Synthetic MRI-aided Pelvic Multi-organ Segmentation in Cone-beam Computed Tomography," Proc. of SPIE, 11313, 1131338-45, 2020.
Fu Y, Lei Y, Liu Y, Wang T, Curran W, Liu T, Patel P and Yang X*. "Cone-beam Computed Tomography (CBCT) and CT Image Registration Aided by CBCT-based Synthetic CT," Proc. of SPIE, 11313, 113132U-9U, 2020.
Fu Y, Lei Y, Zhou J, Wang T, Jani A, Patel P, Mao H, Curran W, Liu T and Yang X*. "Non-rigid MRI-CT Image Registration with Unsupervised Deep Learning-based Deformation Prediction," Proc. of SPIE, 11313, 1131329-36, 2020.
Yang X*, Jani A, Rossi P, Mao H, Curran W and Liu T. “MRI-CT Prostate Registration Using Sparse Representation Technique.” Proc. of SPIE, 978627-978627-8, 2016.
Yang X*, Rossi P, Jani A, Mao H, Ogunleye T, Curran W and Liu T*. “A MR-TRUS Registration Method for Ultrasound-Guided Prostate Interventions.” Proc. of SPIE, 94151Y, 94151Y-9, 2015.