Quantitative cone beam computed tomography (CBCT) imaging is increasing in demand for precise image guided radiation therapy since it provides a foundation for advanced image-guidance techniques, including accurate treatment setup, online tumor and organ delineation and patient dose calculation. With more precise treatment monitoring from accurate CBCT, dose delivery errors can be significantly reduced in each fraction and further compensated for in subsequent fractions using adaptive radiation therapy. However, the current CBCT has severe artifacts mainly due to scatter contamination and its current clinical application is therefore limited to patient setup based on only bony structures.
CBCT-based Synthetic CT
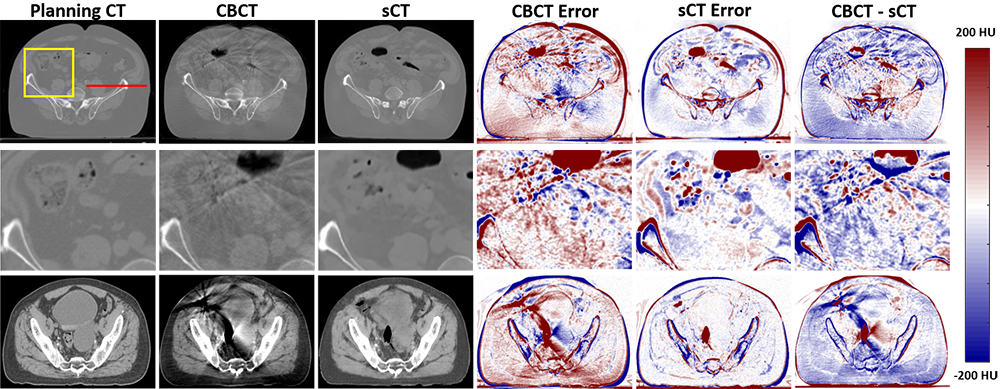
Related publications:
Lei Y, Tang X, Liu T, Jwoong J, Higgins K, Dhabaan A, Wang H, Press R, Curran W and Yang X*. "Learning‐based CBCT Correction Using Alternating Random Forest Based on Auto‐context Model", Medical Physics, 46(2), 601-6182018, 2019. (Selected and Featured in the journal cover and Editor’s Choice)
Harms J, Lei Y, Wang T, Zhang R, Zhou J, Tang X, Curran W, Liu T, and Yang X*. “Paired cycle-GAN-based image correction for quantitative cone-beam computed tomography,” Medical Physics, 46(7), 3325-3337, 2019.
Liu Y, Lei Y, Wang T, Fu Y, Tang X, Curran W, Liu T, Patel P and Yang X*. “CBCT-based Synthetic CT Generation using Self-attention CycleGAN for Pancreatic Adaptive Radiotherapy,” Medical Physics, 47(6):2472-2483, 2020.
Wang T, Lei Y, Manohar N, Tian S, Jani A, Shu H, Higgins K, Dhabaan A, Patel P, Tang X, Liu T, Curran W, and Yang X*. “Dosimetric Study on Learning-Based Cone-Beam CT Correction in Adaptive Radiation Therapy," Medical Dosimetry, 19, 300342, 2019.
CBCT-based Synthetic MRI
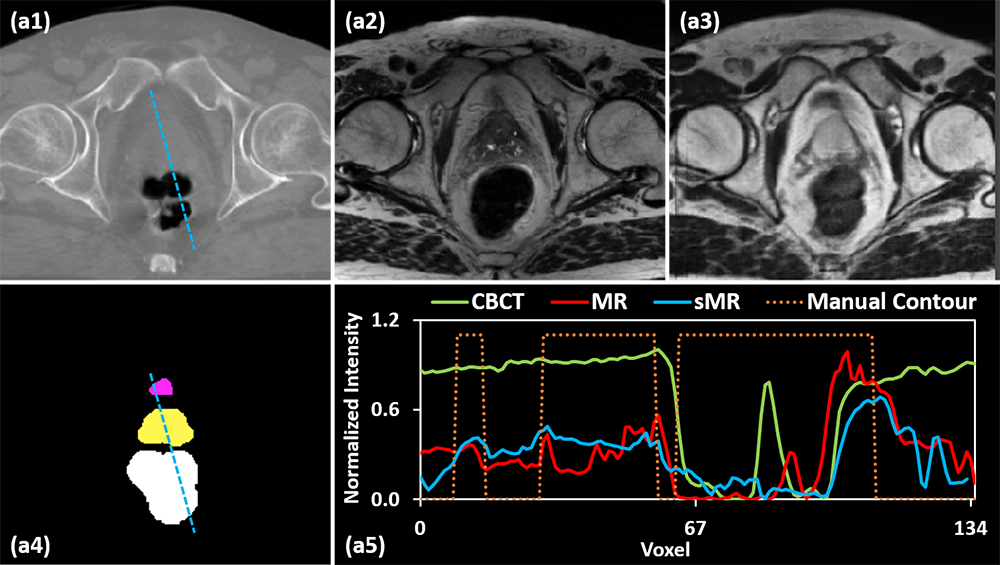
We combined the superior bony structure information provided by CBCTs and the superior soft-tissue information provided by CBCT-based synthetic MRIs via our proposed deep learning networks to simultaneously segment both soft-tissue and bone organs required by prostate treatment planning.
Related publications:
Lei Y, Wang T, Tian S, Dong X, Jani A, Schuster D, Curran W, Patel P, Liu T and Yang X*. "Male Pelvic Multi-Organ Segmentation Aided by CBCT-based Synthetic MRI," Physics in Medicine and Biology, 65(3), 035013, 2020.
Fu Y, Lei Y, Fu Y, Wang T, Tian S, Patel P, Jani A, Curran W, Liu T and Yang X*. “Pelvic Multi-organ Segmentation on CBCT for Prostate Adaptive Radiotherapy," Medical Physics, 47(8):3415-3422, 2020.
Dong X, Lei Y, Liu Y, Wang T, Tian S, Jani A, Patel P, Shuster D, Curran W, Liu T and Yang X*. "Synthetic MRI-aided multi-organ segmentation on male pelvic CT using cycle consistent deep attention network," Radiotherapy and Oncology, 141, 192-199 2019.
Lei Y, Dong X, Tian Z, Liu Y, Wang T, Tian S, Patel P, Jani A, Curran W, Mao H, Liu T and Yang X*. "CT Prostate Segmentation Based on Synthetic MRI-aided Deep Attention Fully Convolution Network," Medical Physics, 47(1), 530-540, 2020.
CBCT-based SPR Mapping
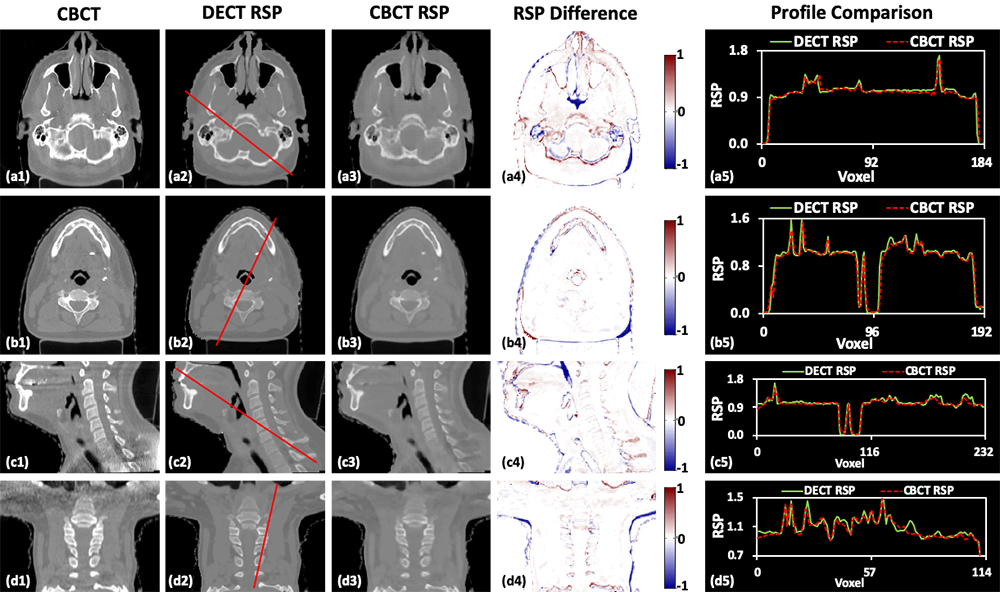
Compared to helical CTs, CBCT have less accurate Hounsfield Units and degrade image quality, limiting their potential use for proton dose calculation. A deep-learning-based approach is developed to accurately estimate relative stopping power (RSP) from to daily CBCTs, to enable future CBCT-guided online proton dose evaluation and adaptive proton planning.
Related publications:
Harms J, Lei Y, Wang T, Ghavidel B, Stokers W, McDonald M, Curran W, Liu T, Zhou J and Yang X*. “Cone-beam CT-derived relative stopping power map generation via deep learning for adaptive proton radiotherapy," Medical Physics, 47(9), 4416-4427, 2020.
Wang T, Harms J, Lei Y, Ghavidel B, Stokes W, Liu T, Curran W, McDonald M, Zhou J and Yang X*. "Deep Learning-Based Relative Stopping Power Mapping Generation with Cone-Beam CT in Proton Radiation Therapy," Proc. of SPIE, 11312, 113124L-30L, 2020.