Positron emission tomography (PET) has been used as a non-invasive functional imaging modality with a wide range of clinical applications. Recently, with the focus shifting towards precision medicine, it is of great clinical interest in accurate quantitative measurement on tracer uptake, where the role of PET is expanded to more demanding applications such as estimation of target occupancy in drug development, therapeutic response monitoring, and treatment outcome prediction as a prognostic factor. The accuracy of the uptake quantification, on which these potential applications rely, would be limited due in part to the physical nonidealities including photon attenuation, low count statistics, partial-volume effect and etc. These issues result in bias, uncertainty and artifacts on the PET images, which would degrade the utility of both qualitative and quantitative assessments. They are becoming conspicuous with the introduction of advanced PET scanners and applications in the recent years, such as MR-combined PET (PET/MR), low-count scanning protocol and high-resolution PET. These novel PET technologies aim to incorporate anatomical imaging modality for better soft tissue visualization, reduce administered activity and shorten scan time of conventional PET and PET/MRI, and increase detection capability on radiopharmaceutical accumulation in structures of millimeter size, all of which are highly desirable for clinical practice.
Related publications:
Wang T, Lei Y, Fu Y, Curran W, Liu T and Yang X*. “Machine Learning in Quantitative PET: A Review of Attenuation Correction and Low-count Image Reconstruction Methods,” European Journal of Medical Physics (Physica Medica), 76, 294-306, 2020.
PET AC in PET/MRI Scanner
Deriving accurate attenuation maps for PET/MRI are challenging due to common problems, such as truncation, inter-scan motion, and erroneous transformation of structural voxel-intensities to PET µ-map values. Methods employing template- and atlas-based segmentation can introduce non-patient specific features related to registration errors and interpatient anatomical variability. We present a serial of learning-based AC methods including Self-PET AC, PET-based synthetic CT, MRI-based synthetic CT and MRI-aided PET AC.
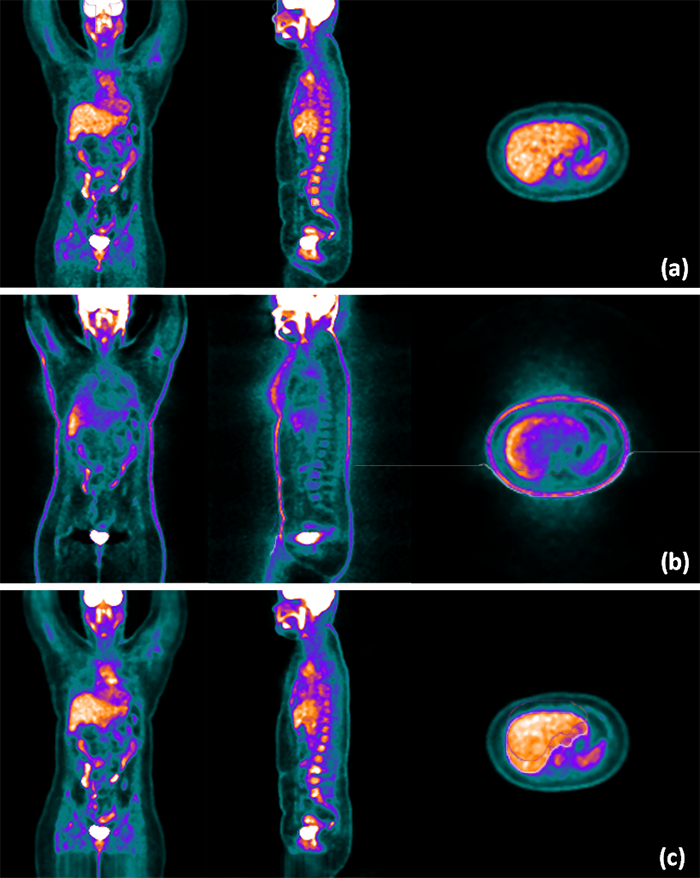
Self-PET AC
Related publications:
Dong X, Lei Y, Wang T, Higgins K, Liu T, Curran W, Mao H, Nye J and Yang X*. “Deep Learning-based Attenuation Correction in the Absence of Structural Information for Whole-body PET imaging,” Physics in Medicine and Biology, 65 (5), 036015, 2020. (Jack Krohmer Junior Investigator Competition Winner (1/174) at annual meeting of AAPM in 2019)
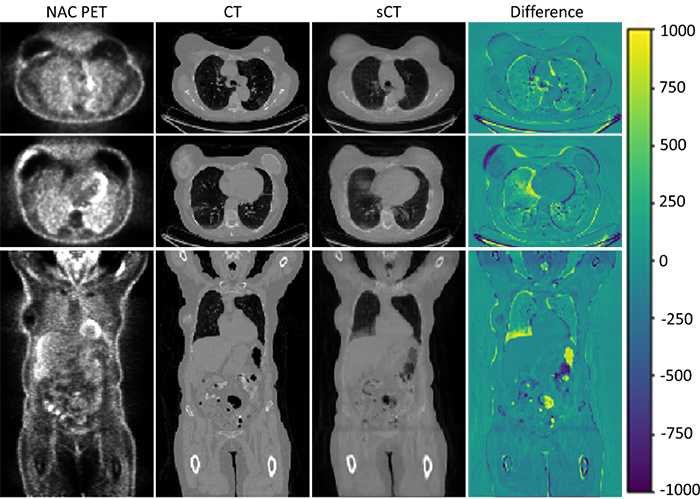
PET-based synthetic CT for PET AC
Related publications:
Dong X, Lei Y, Wang T, Higgins K, Liu T, Curran W, Mao H, Nye J and Yang X*. “Synthetic CT generation from non-attenuation corrected PET images for whole-body PET imaging,” Physics in Medicine and Biology, 64(21): 215016, 2019.
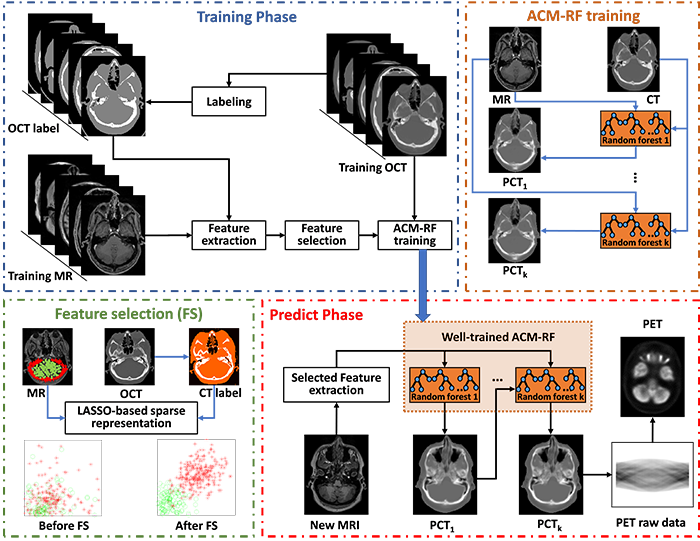
MRI-based synthetic CT for PET AC
Related publications:
Yang X*, Wang T, Lei Y, Kristin H, Liu T, Shim H, Curran W, Mao H, and Jonathon N. " MRI-based Attenuation Correction for Brain PET/MRI based on Anatomic Signature and Machine Learning", Physics in Medicine and Biology, 64 (2), 025001, 2019.
Lei Y, Harms J, Wang T, Liu Y, Shu H, Jani A, Curran W, Mao H, Liu T and Yang X*. “MRI-Only Based Synthetic CT Generation Using Dense Cycle Consistent Generative Adversarial Networks,” Medical Physics, 46(8), 3565-3583, 2019. (Selected and featured on the journal cover of Medical Physics)
Lei Y, Harms J, Wang H, Tian S, Zhou J, Shu H, Zhong J, Mao H, Curran W, Liu T and Yang X*. “MRI-based Synthetic CT Generation Using Semantic Random Forest with Iterative Refinement," Physics in Medicine and Biology, 5, 64(8):085001. 2019.
Lei Y, Shu H, Liu T, Mao H, Shim H, Curran W and Yang X*. "A Leaning-based Approach to Derive Electron Density from MRI Using Random Forest and Iterative Refinement Model", Journal of Medical Imaging, 5(4), 043504, 2018.
Lei Y, Shu H, Liu T, Shim H, Mao H, Tian S, Jeong J, Jani A, Curran W and Yang X*. "MRI-based Pseudo CT using Anatomic Signature and Jointly Dictionary Learning", Journal of Medical Imaging, 5(3), 034001, 2018.
Yang X, Lei Y, Shu HK, Rossi P, Mao H, Shim H, Curran WJ, Liu T. Pseudo CT Estimation from MRI Using Patch-based Random Forest. Proc. of SPIE. 10133:101332Q, 2017. doi: 10.1117/12.2253936. PMID: 31607771; PMCID: PMC6788808.
Yang X and Fei B. "Multiscale Segmentation of the Skull in MR Images for MRI-based Attenuation Correction of Combined MR/PET." Journal of the American Medical Informatics Association, 20(6), 1037-1045, 2013.
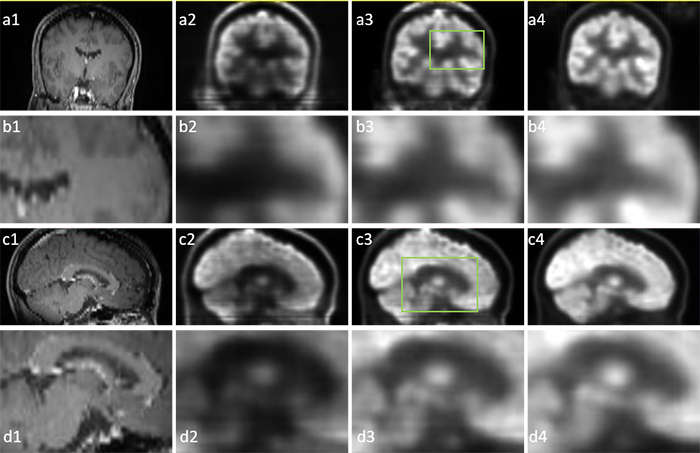
MRI-aided PET AC
Related publications:
Lei Y, Dong X, Wang T, Higgins K, Liu T, Curran W, Mao H, Nye J and Yang X*. "MRI-aided Attenuation Correction for PET imaging with Deep Learning," Proc. of SPIE, 11317, 1131723-29, 2020.
Low-Dose PET Imaging
Lowering either the administered activity or scan time is desirable in PET imaging as it decreases the patient's radiation burden or improves patient comfort and reduces motion artifacts. But reducing these parameters lowers overall photon counts and increases noise, adversely impacting image contrast and quantification. To address this low count statistics problem, we propose two deep-learning-based methods including self low-dose PET imaging and CT-aided low-dose PET imaging.
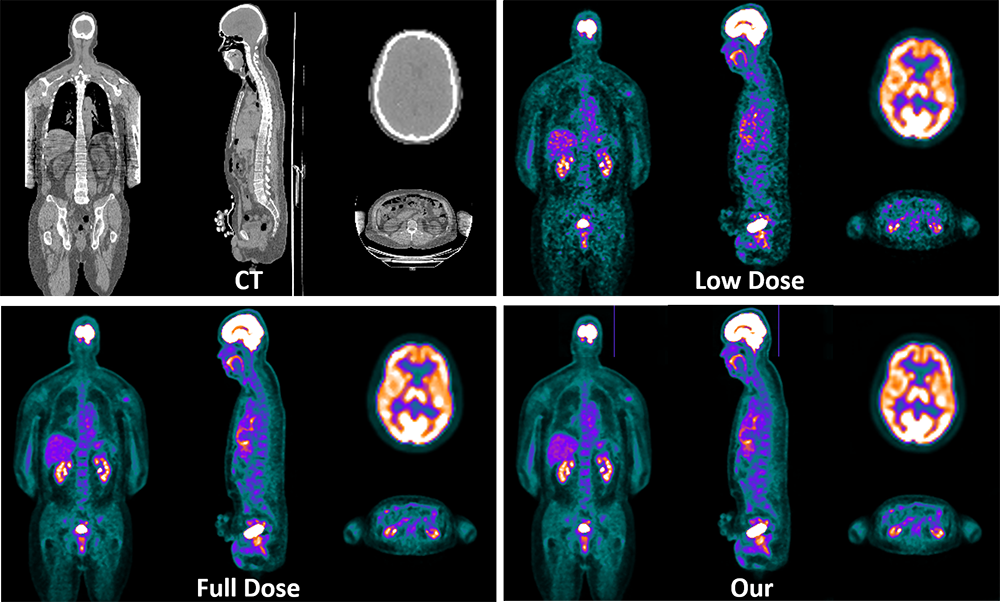
Self low-dose PET imaging
Related publications:
Lei Y, Dong X, Wang T, Higgins K, Liu T, Curran W, Mao H, Nye J and Yang X*. “Whole-body PET Estimation from Low Count Statistics using 3D Cycle-Consistent Generative Adversarial Networks,” Physics in Medicine and Biology, 64(21): 215017, 2019.
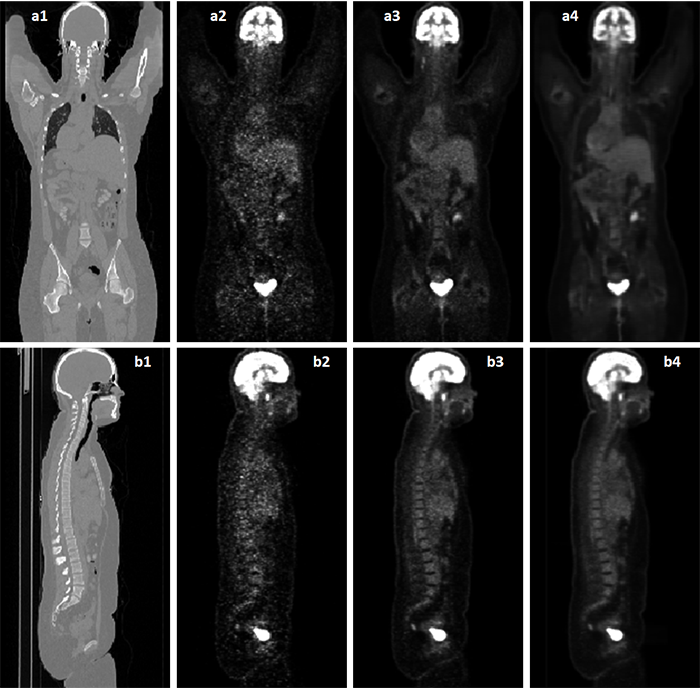
CT-aided low-dose PET
Related publications:
Dong X, Lei Y, Wang T, Higgins K, Liu T, Curran W, Mao H, Nye J and Yang X*. "Low Dose PET Imaging Based with CT-aided Cycle-consistent Adversarial Networks," Proc. of SPIE, 11312, 1131247-54, 2020.
Fast and High-Resolution MR Imaging
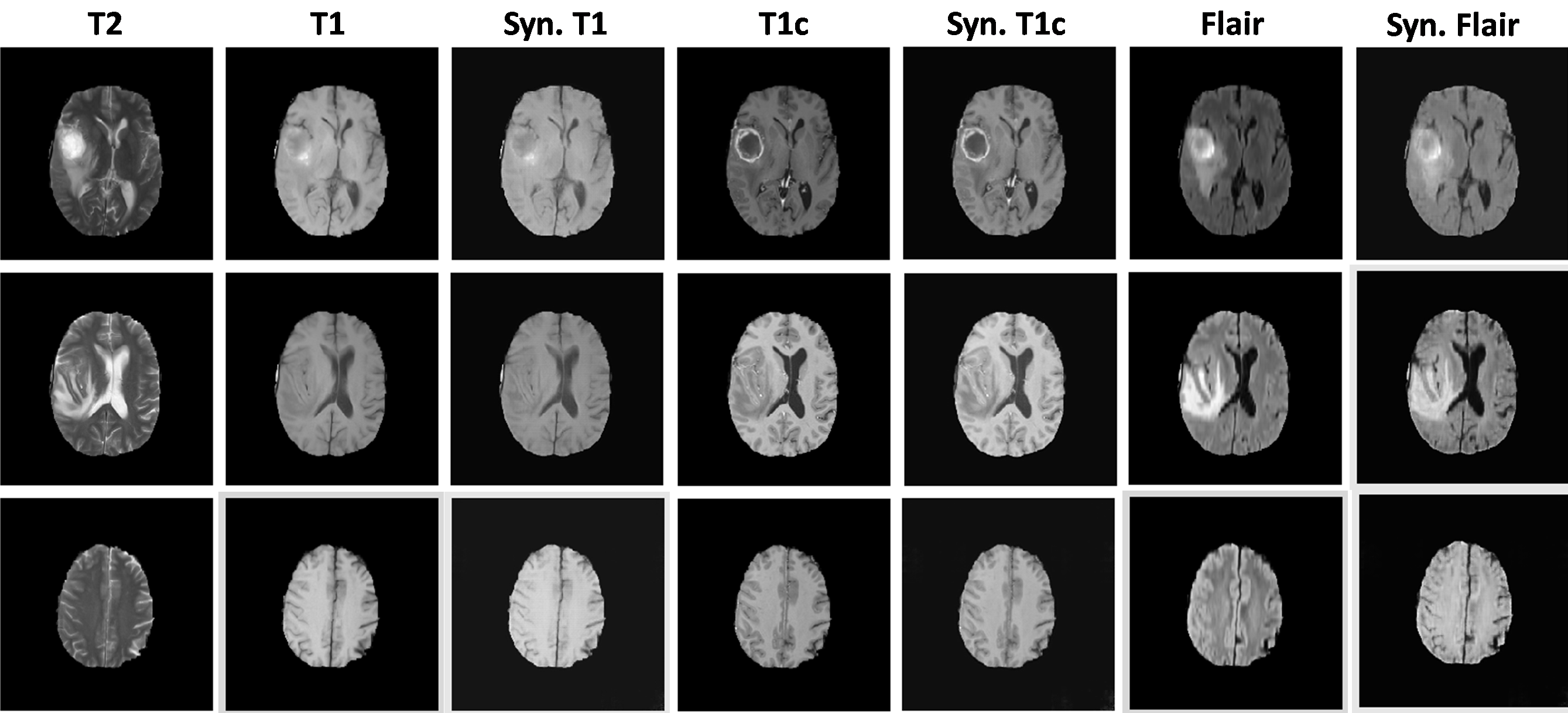
Related publications:
Dai X, Lei Y, Fu Y, Curran W, Liu T, Mao H and Yang X*. "Multimodal MRI Synthesis Using Unified Generative Adversarial Networks," Medical Physics, 47(12):6343-6354, 2020.
He X, Lei Y, Fu Y, Mao H, Curran W, Liu T* and Yang X*. "Super-Resolution Magnetic Resonance Imaging Reconstruction Using Deep Attention Networks," Proc. of SPIE, 11313, 113132J, 2020.