The Satten Lab develops novel statistical methods that can be used to analyze data from Microbiome studies; develops methods to analyze data from epidemiological studies, especially studies in genetic epidemiology; and applies these and other methods to studies of reproductive health.
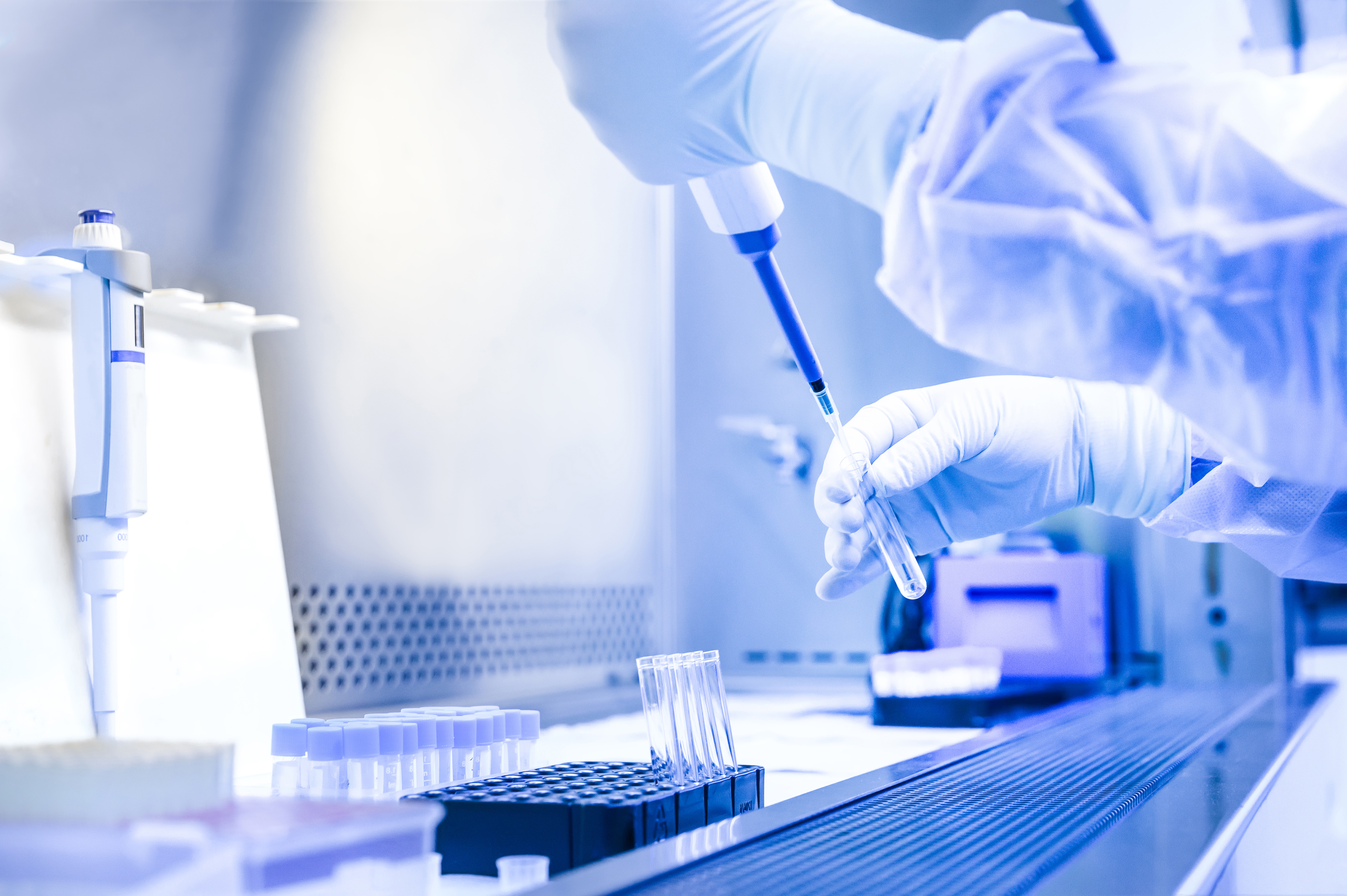
Our research centers on how to best analyze data from association studies involving the microbiome. Many of these studies are complex, and involve matching or other forms of clustering, longitudinal data or survival outcomes. Furthermore, microbiome data are subject to bias at every step of the analysis pipeline; our lab has a special interest in methods that properly account for these biases. Finally, general questions in epidemiology (especially genetic epidemiology) such as mediation analysis are always of interest.
Collaborators (Faculty/CDC)
- Yijuan Hu (Emory RSPH)
- Ni Zhao (Johns Hopkins BSPH)
- Wodan Ling (Cornell-Weil)
- Anne Dunlop (Emory Gyn-OB)
- Charlan Kroelinger (CDC)
Current Lab Members (Students)
- Jiuyao Lu (Johns Hopkins SPH)
- Mengyu He (Emory SPH)
- Ye Yue (Emory SPH)
Former Lab Members
- Yingtian Hu (Emory SPH)
- Yunxiao Li (Emory SPH)