Artificial Intelligence Development, Validation, and Implementation

Artificial intelligence is one of the fastest growing innovations in medical imaging and offers great potential to improve patient care. However, for the medical field to optimally benefit from this development, AI algorithms should be developed in close collaboration with AI and medical experts. It is essential that these application are clinically validated and optimized for clinical work flow integration and implementation. Our group collaborates with several partners, including universities, industry partners and medical specialist, to optimize the clinical development and use if AI application for cardiothoracic imaging purposes.

COVID-19 Severity Classification and Prognostication
In collaboration with colleagues in the School of Electrical and Computer Engineering of the Georgia Institute of Technology, we are developing an accurate and automated approach based on artificial intelligence (AI) for prognosis of COVID-19 pneumonia using imaging, clinical, and demographical data.
We’ve built computer neural networks that use chest X-rays to classify disease severity, using multi-reader labeling, taking into account clinical reader variability. Analysis of influencing clinical factors on disease severity, progression, and outcome is of great importance, given the high volume of data, which makes this impractical in clinical studies.
Using interpretable models for understanding such hidden patterns in the high-dimensional data that are not visible to human experts can help study the relation between different clinical and imaging parameters and find the most influential features on disease progression and outcome. With this approach we try predict disease progression and outcome for individual patients.
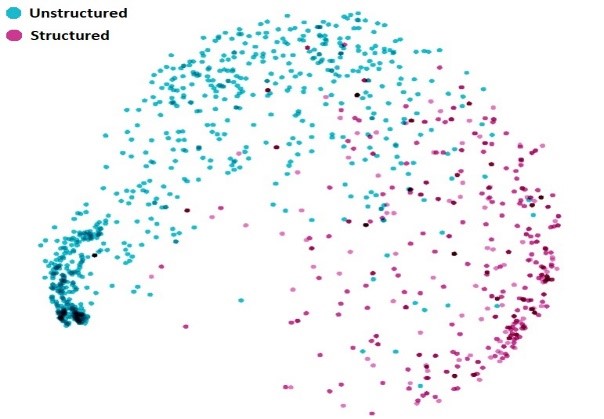
Fusion Artificial Intelligence Model Combining Cardiac CT Imaging and Electronic Medical Records Data to CAD-RADS and Outcome
Data from non-invasive imaging techniques allowing direct assessment of coronary atherosclerosis can provide significant incremental value to clinical data for outcome prediction. The Coronary Artery Disease Reporting and Data System (CAD-RADS) is a standardized reporting system developed for coronary computed tomography angiography (CCTA). CAD-RADS is related to the severity of coronaray artery disease (CAD), and helps to standardize CAD diagnosis and patient management.
We develop an automated and efficient clinical approach based on fusion artificial intelligence, combining electronic medical record (EMR) data and imaging data from coronary artery calcium score (CAC) and CCTA, to accurately predict clinical outcomes in patients with coronary artery disease. We developed an algorithm aimed at bridging the "gap" between structured and unstructured reporting using natural language processing (NLP).
Quantification of Cardiothoracic Imaging Biomarkers
With the increase in technological abilities in the field of medical imaging, we see an increasing role or quantitative imaging. Quantitative imaging reduces the variability and can improve early detection of disease, the diagnosis and severity classification and assist in follow-up and prognostication. We use several advanced analysis approaches, including CT derived FFR, myocardial blood flow quantification and dual energy based iodine quantification to advance the field of cardiothoracic imaging.
MyocardiAI CT Perfusion and Coronary Flow: a CompreHensive Cardiac CT Myocardial Perfusion Imaging (MPI)/Franctional Flow Reserve (FFR) and PET-CT MPI Evaluation (The MATCH Investigation)
In this study we aim to evaluate absolute quantification of myocardial perfusion done by CT myocardial perfusion imaging (CT-MPI) and the coronary flow measured by CT Fractional Flow Reserve analysis (CT-FFR) compared to the gold standard represented by PET myocardial perfusion imaging (PET-MPI).
Novel Approaches to Cardiothoracic Imaging
With the advances in hardware technology, novel imaging protocols become available for more advanced imaging. Especially for cardiac imaging, traditionally cardiac imaging is challenging because of the technological requirements that are needed to deal with cardiac motion and the size of some structures, such as coronary arteries. Our group experiments with the development of these protocols. As part of Emory’s continued commitment to driving discovery through investment in technology and infrastructure, Emory has recently installed a PET/MR system and a 7 Tesla MRI. Research will be focused on optimizing these systems for state of the art (multi-modality) imaging.
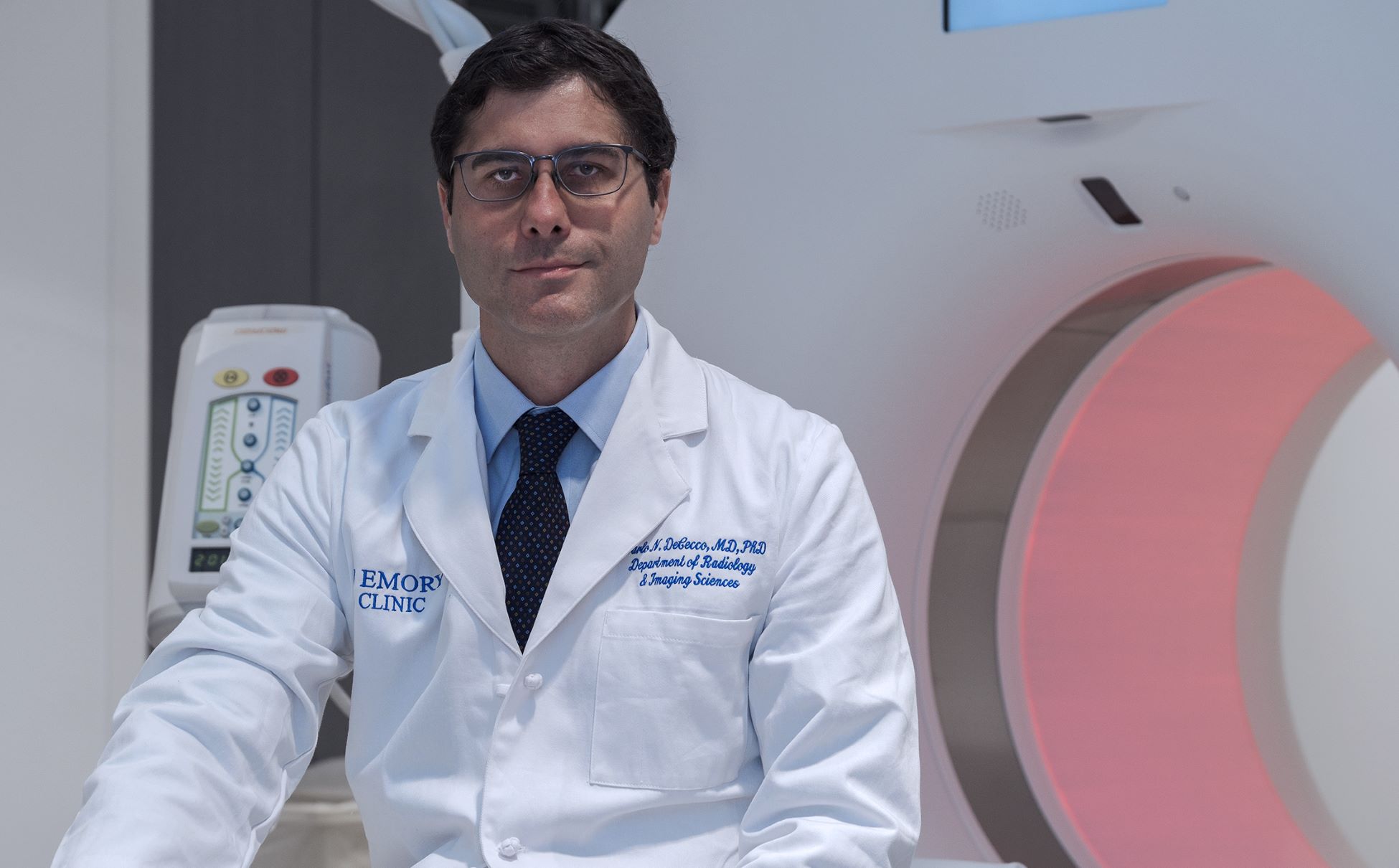